2D Materials as 'winner-takes-all; neurons for next-generation AI computing
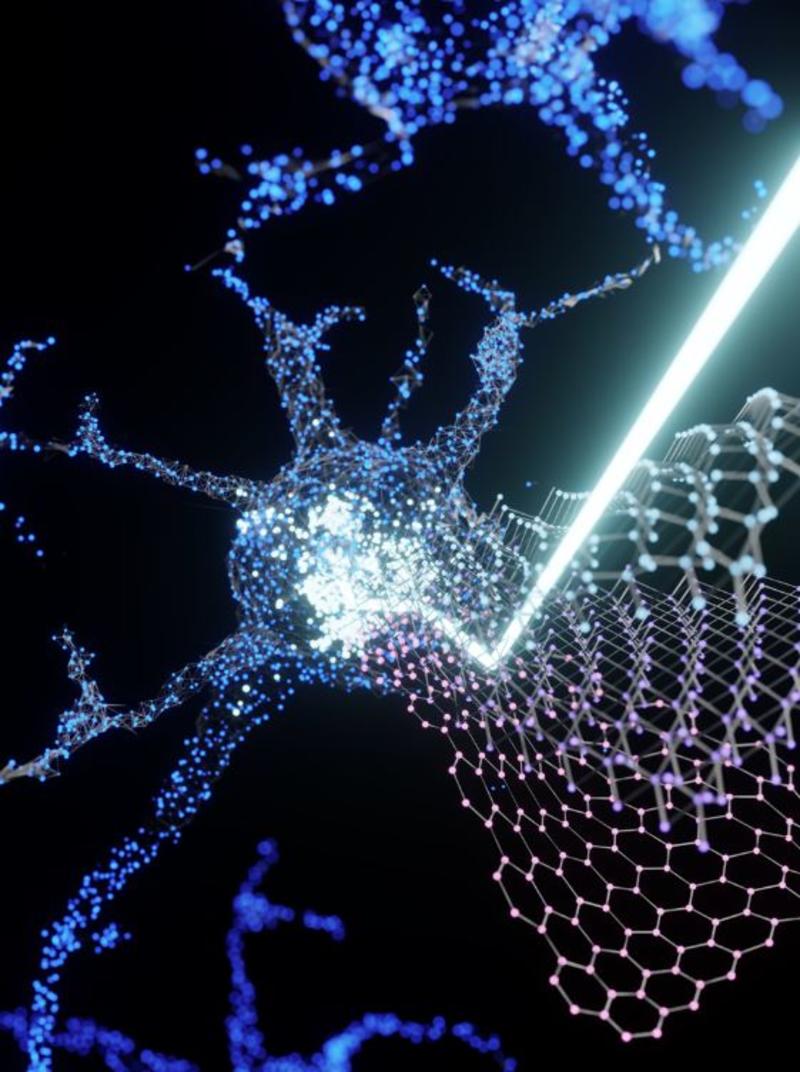
"This entire field is super exciting, as materials innovations and novel insights into how they can be creatively applied all need to come together, and this work represents a new toolkit, exploring the power of two-dimensional materials, not in transistors, but for novel computing paradigms".
Professor Harish Bhaskaran
New research uses a stack of 2-dimensional (2-D) materials to create neurons using both light and electric signals for computing.
For decades, scientists have been considering the possibility of recreating the versatile computational capabilities of biological neurons to develop faster and more energy-efficient machine learning systems. One promising approach in this endeavour involves the use of memristors, which are electronic components capable of storing a value by modifying their conductance and then utilising that value for in-memory processing.
Despite their potential to increase the speed and efficiency of machine learning, electronic memristors and the neural networks created from them still have a long way to go before they can replicate the complex processes of biological neurons and brains. This is particularly true for mechanisms that integrate feedforward and feedback neuronal signals. As an example, such mechanisms underpin our cognitive ability to learn complex tasks, using rewards and errors.
In a paper* published in Nature Nanotechnology on 4 May, researchers at the University of Oxford, IBM Research Europe, Denmark Technical University and the University of Texas at Austin, have developed atomically thin artificial neurons by stacking 2-D materials. The researchers integrated optical signals into the electronic memristors to expand their functionality, enabling the existence of separate feedforward and feedback paths. This advancement allowed the team to create winner-takes-all networks, which demonstrated the potential for solving complex computational problems in machine learning, such as unsupervised learning in clustering and combinatorial optimisation problems.
2-D materials are made up of just a few layers of atoms, and theyhave some exotic properties because of these size scales. These properties can be fine-tuned by layering them with each other judiciously. Dr Ghazi Sarwat Syed, the lead author of the study and a Research Staff Member at IBM Research Switzerland, explains that their devices are composed of a stack of three 2-D materials - Graphene, Molybdenum disulfide and tungsten disulfide. Unlike digittal storage devices, these devices are analog and operate similarly to the synapses and neurons in our biological brain. The analog feature allows for computatations, where a sequence of electrical or optical signals sent to the device produce produces gradual changes in the amount of stored electronic charge. This process forms the basis for threshold modes for neuronal computations, which is analogous to the way our brain processes a combination of excitatory and inhibitory signals when it computes through schemes such as winner-takes-all.
'This is a highly exciting development. This paper is the culmination of years of experimental and modeling work, as well as extensive analysis and consideration of the problems that such systems can address. These problems are highly relevant as we explore the potential applications and algorithms of AI hardware and neuromorphic engineering '.
Dr Gyed Sarwat Syed
Dr Syed and Dr Yingqiu Zhou (who were DPhil students and lab colleagues at Oxford) conducted the experimental work. According to Dr Zhou (a postdoctoral researcher at Denmark Technical University) their implementation captures the essential components of a biological neuron through the optoelectronic physics of low-dimensional systems. She says:
'We have created an atomically abrupt heterojunction through the design of our heterostructure stack. The stack, therefore, delivers a heterojunction that acts as the neuronal membrane, while the graphene electrodes that contact the heterojunction serve as the neuronal soma. This way the neuronal state is presented in the soma, but modified by the changes in the membrane, just like in actual neurons'.
Dr Yingqiu Zhou
As computing for artificial intelligence applications has taken off, the computational power required is growing at a much faster rate than Moore's Law; new hardware has not kept pace with the dizzying innovations in software, and the computational power and load of current day processors simply cannot grow. New techniques are being researched including in the lead author Harish Bhaskaran's Advanced Nanoscale Engineering Laboratory.
In collaboration with Professor Jamie Warner (University of Texas at Austin) the research team developed the device and also carried out modeling to validate some of the hypothesis for why such a layered device works. Professor Warner was able to tell us that:
'The use of such 2-D structures in computing has been spoken about for years, but the fact that this is a new area of computing allows us to finally realise the potential of such layered materials, so called "heterostructures". We are finally seeing the pay-off after spending more than seven years in developing the synthesis and assembly of wafer-scale 2-D monolayers into complex ultrathin opto-electronic devices and the start of new information processing approaches that is using 2-D materials based on industrially scalable fabrication methods".
Professor Jamie Warner
The findings are more of an exploratory nature than actual demonstrations at the system level, Dr Syed and Professor Bhaskaran point out. In their recent publication* they introduce a novel concept that surpasses the fixed feedforward operation typically utilised in current artificial neural networks. Although they aim to expand on the concept in the future, they are convinced that their current proof-of-principle results demonstrate an important scientific interest in the wider fields of neuromorphic engineering, enabling them to better emulate and comprehend the brain. Exciting research developments are important for future innovation, but this is not technology that one should expect in their mobile phones during the next two years.
* You can read the full paper online: 'Atomically thin optomemristive feedback neurons'.